Positive Deviance Goes Viral: Observations From the WirVsVirus Hackathon
April 14, 2020
Marie-Helen Cymorek, Andreas Glücker, Valentin Kruspel
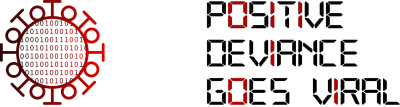
Doing Our Part: A Community-Based Response to a Global Challenge
Within a matter of weeks, the COVID-19 pandemic has changed lives around the globe, disrupting the social, economic, and political landscape. In-person social interactions are constrained while large social events, meetings, and parties are strongly discouraged. On a global and local level, new challenges emerge daily, the scope and magnitude of which reveal some of our greatest societal vulnerabilities; what’s more, past solutions no longer apply within the current context.
Instead of falling into apathy amid these challenges, individuals, groups, communities, and whole societies around the globe have come together to support each other, developing novel solutions to unexpected challenges. For example, around 42.000 volunteers (almost 30.000 working night shifts) helped to develop digital solutions for the COVID-19 crisis in context of the WirVsVirus hackathon - from recording the transmission of new cases and supporting the mental health of people in quarantine to digitizing public administration services.
The GIZ Data Lab wanted to do our part, so we developed an idea to combine and analyze various kinds of available public data in an attempt to identify those communities that are performing unexpectedly well during the COVID-19 outbreak. We believe there are communities managing to adapt significantly faster and better to the outbreak than others, thereby reducing negative outcomes. We call these communities “Positive Deviants.”
Data-Powered Positive Deviance in the Context of Pandemic
The idea of Positive Deviants is grounded in the assumption that, within every community or organization, there are a few individuals or groups who develop highly adaptive solutions that enable them to outperform their peers. This approach can be applied in virtually any context where performance depends on not only access to resources or structural conditions but also on the behavior of individuals. The beauty of this approach is that such solutions do not require a lot of resources and are developed by the communities themselves, making them incredibly effective, highly context-sensitive, and usually given a high level of ownership by their communities (Pascale, Sternin and Sternin, 2010).
Albanna and Heeks (2018) took this approach one step further, lifting it into the digital age. While the traditional approach required extensive resource and time-intensive field research to find Positive Deviants and understand their solutions, these researchers found that Positive Deviants can also be discovered using digital data. At the GIZ Data Lab, we aim to use their approach to accelerate the identification of local solutions that could empower hundreds of communities around the globe.
Within the context of the COVID-19 pandemic, our GIZ Data Lab team realized the Positive Deviance approach had great potential to help counties and municipalities deal with unprecedented challenges. According to this method, we felt convinced that there must exist certain communities that are successful in dealing with the consequences of a viral outbreak, even while facing similar circumstances to all others. During the WirVsVirus hackathon, we inspired about 40 people from varying professional backgrounds to test Positive Deviance in this context. Relying on a combination of different skills, talents, and perspectives we developed the first data-based analysis with a speed and quality that none of us could have achieved on our own. The dynamics and efficiency of brief, intensive cooperation fully convinced us that this approach can contribute to better insights and strategies in dealing with COVID-19.
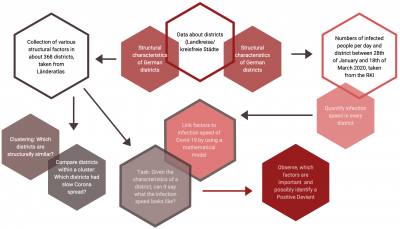
Methodology, Approach, and Future Applications
Much of our work throughout the hackathon centered on data collection, with a focus on structural factors such as the number of doctors, hospitals, academic workers, or unemployment ratio - all information we retrieved from sources like Landatlas or Wikipedia. Soon, we hope to gain additional insights into how individual behavior accelerates or slows the spread of COVID-19. We expect that, over the course of the crisis, more data will become available, making us better able to quantify and investigate critical behavior.
As we endeavored to identify deviant communities, comparability is key. We statistically controlled for a wide range of structural variables, such as population density, hospital beds, or age distribution. Then, we were able to cluster counties and municipalities with similar resources and structural conditions. Based on an analysis of these clusters, we might someday be able to identify structural conditions that correlate with the spread of the virus. However, our focus lay on the identification of those communities outperforming others in managing COVID-19 independent from accessible resources or other structural conditions - that is, as a result of specific behavior and solutions.
From a methodological perspective, we aimed to develop a machine-learning model that can unveil links between district-specific structural features and the infection rate of COVID-19. We believe that, with further precision and training, the model can act much like a crystal ball in identifying the “rules” connecting structural factors to infection rate. This can serve as the basis for seeking out positive deviants and their unique behavior.
Next Steps and First Impressions
Based on gathered data and our first analysis throughout the hackathon, we were able to gain insight into the impact of structural variables on the spread or slowing of the COVID-19 pandemic. In order to document our learnings, we built a website that visually captures our initial results of both data clusters and the application of machine learning techniques.
We feel inspired to continue our progress in the coming weeks and months. Here, we’d like to share impressions from some of our hackathon team members - now new Positive Deviance Enthusiasts - to help inspire you as well:
-
Elena Leuschner
“I had never heard of Positive Deviance before. But the idea of identifying best-cases and examining their behavioral patterns sounded immediately interesting to me. Combining an approach that is rooted in psychology and global studies with digital data and machine-learning methodologies enabled us to analyze the COVID-19 crisis from a totally different angle. One of the main challenges that we faced as a group was successful communication. We were about 40 people with different areas of expertise. I am extremely proud that our outcome is indeed the result of joint work and effort. Without the input of every team member, this experience wouldn’t have been the same.”
-
Bart Vollebergh
“I jumped into the project at the encouragement of some friends from university and contributed in multiple ways - especially with regards to project design and documentation. For me, searching for interesting and reliable data sources felt a bit like treasure hunting – it was fast and challenging but also exciting. I believe that the Positive Deviance approach will become even more feasible as more data becomes available. From my perspective, the isolation of factors responsible for deviant behaviors promises to bear results that can be implemented across local contexts and therefore account for the global scale of a pandemic.”
-
Clara Kuper
"I experienced the hackathon as very fast-paced and intense. We organized ourselves in different teams with different tasks - for example, the collecting, merging, and cleansing of data – but still managed to work together. One thing I enjoyed most was our regular update calls. It was interesting to see how different approaches to the challenge popped up and how the team managed to keep different workflows aligned. Personally, I tried to identify proxies for deviant behaviors by using Twitter data. I used R, Python and a twitterscraper from GitHub to collect COVID-19 related tweets in the German language. Forty-eight hours was not enough time to finish my project, but as I believe that our approach still lacks some behavioral input, I’ll keep tinkering on my script.”
-
Charlotte Felbinger
“What stood out most to me during the challenge was how much social scientists and data analysts need each other - a theoretical concept needs reliable data and adequate models to show results; at the same time, data needs theoretical guidance to produce constructive outcomes. In my academic environment, I hear many people preaching interdisciplinary. However, from my own experience, good interdisciplinary work is not easily done. Joining forces and producing tangible outcomes in such a short time frame was a particular challenge - one which we managed to accomplish!”
-
Fynn Withake
“Personally, I love how the data-based Positive Deviance approach unites technical aspects like programming and mathematics with relevant questions from sociology. In the context of this challenge, it was very rewarding to participate in so many different tasks. During the first period of the project, my contribution was mainly collecting structural data and researching for (at that time not-yet-existing) statistics about the numbers of conducted COVID-19 tests in Germany. On the second day, I set up the website using Bootstrap and GitHub Pages, which turned out to be a good choice for rapid prototypes. Overall, I was really impressed by the skills and expertise of my team members, many of whom were professionally involved in some data science-related work. The general attitude of the people involved in this project was awesome, and I’m really happy with the outcome.”